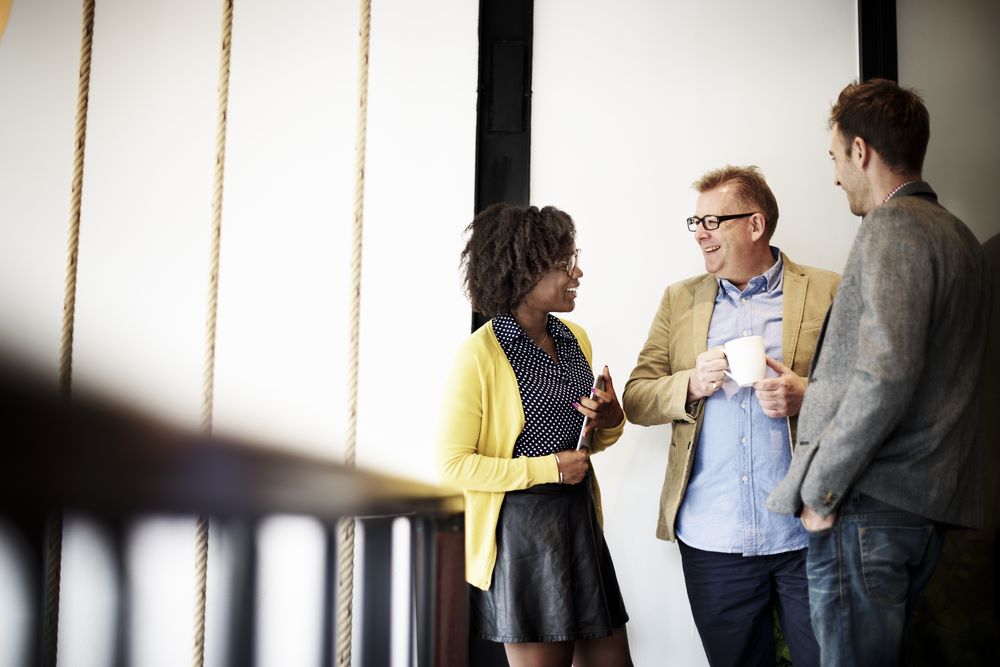
The Importance of Communication for Data Science and Analysis
Data Science is often viewed as a purely technical discipline that encompasses all kinds of mind-boggling mathematics, software development skills and domain knowledge. Often Data Scientists will focus their learning and development areas in these three disciplines and neglect to give the development of their soft skills the same attention.
Perhaps the most important, and most neglected soft skill of all is communication. After all, if you can’t adequately explain your work to your peers and more importantly senior leaders, organisations will not act upon your information. At Mango we see these issues all the time, and this was partly the inspiration for our Trusted Consultant training course which encompasses planning, organisation and you guessed it, communication.
In this blog we’ll look at a few simple approaches that we teach in our trusted consultant course that can improve communication skills.
Keep Your Audience in Mind
The mistake we see most often is not keeping the audience in mind, both in terms of technical ability and in terms of what they’re most interested in. This is especially true of presentations.
A good example of this is senior leadership. They’re most interested in the bottom line… How data and data science can unlock the potential in an organisation, most often through improving efficiency and increasing profitability. Senior leaders don’t want to sit through formulae, complex charts and detailed explanations. They care most about impact, so a presentation to them should be tailored toward this, and ideally the outcome should be at the very start of a presentation and not the very end.
Do the Simple Things Well
As with most things in life, 80% of the battle with communication, is doing simple things well and this is especially true when it comes to charts. As a base rule every chart should have:
- Title
- Axis labels
- Legend / key
- Dates / Time period (if appropriate)
- Data Source
A little effort with the design can also make a big difference, particularly through emphasis. The most common thing people do is more place emphasis on more important aspects of a chart (e.g. the title) through increased font size or bold . This can work, but in many cases, it’s better to de-emphasise less important aspects of the chart instead.
A good technique here is to colour less important chart aspects in a shade of grey instead of black, particularly the axis and gridlines. Less is sometimes more.
Lastly, your presentation may also be scrutinised without you there to present it. It’s a good idea to provide a little narrative on the slide itself to make sure that it ‘travels well’ and can reach and influence a wider audience than you first presented it to.
Be Memorable
Being memorable isn’t necessarily about organising a pyrotechnic display to accompany your presentation (that would probably make it memorable for all the wrong reasons) but ensuring that your message resonates with your audience so that they understand it and take it away.
A very simple way to do this is using the Rule of Three. If you think about it, three is a very memorable number and we tend to remember things more so in threes than twos or fours. For example:
- The Good, the Bad and the Ugly
- I came, I saw, I conquered
- Blood, Sweat and Tears
You can applythe rule of three in a variety of ways, from splitting your presentation structure into three, by picking the three strongest supporting arguments alongside a proposal, or through summarizing the contents of your presentation into three key takeaways.
Lastly, The more keenly eyed of you will notice that I’ve picked three things to write about as part of this blog. That’s not a coincidence!
In closing
These techniques and more, are covered in detail as part of our Trusted Consultant training material. You can find our course and a summary of what it will cover on our course list page within our Training pages here.
Author – Tom Ewing
Tom is a Senior Data Scientist at Mango Solutions. He’s been a data scientist for 5 years and has spent the majority of his career in a variety of data, statistical and analytical roles before making the jump to data science.